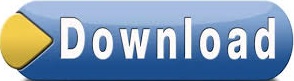
Machine learning algorithms are only as good as the data they are trained on. 50 Open Source Image Datasets for Computer Vision for Every Use Case. As part of our study, we drastically boost the performance of previously proposed techniques and outperform previously published state-of. This reflects the fact that the data provided to the algorithm will determine what patterns the algorithm learns, and thus what content it may correctly recognize in the future.We challenge a number of common practices in selfsupervised visual representation learning and observe that standard recipes for CNN design do not always translate to self-supervised representation learning. Our method also won the 1st place in the DeepFashion2 Challenge 2020 - Clothes Landmark Estimation Track with an AP of 0.590 on the test set, and 0.615 on the validation set.50 Open Source Image Datasets for Computer Vision for Every Use Case. 25.Vision of Humanity | Destination for Peace Extensive experiments show that our method outperforms current state-of-the-art methods by a large margin. Ge et al., DeepFashion2: A Versatile Benchmark for Detection, Pose Estimation, Segmentation and Re-Identification of Clothing Images, In Proceedings of the IEEE Conference on Computer Vision and Pattern Recognition, 2019, pp.5337-5345. A collection of awesome resources clothed people including human digitalization, virtual try-on, and other related directions. This paper is one ofLotayou / awesome-clothed-human. Having automatic item descriptions is of great interest for fashion web platforms hosting sometimes hundreds of thousands of images. Image captioning has increasingly large domains of application, and fashion is not an exception. The particular focus is on facial landmark detection in real-world datasets of facial images captured in-the-wild. You will have to predict a probability of having at least one claim over the insured period of a building.The DeepFashion2 challenge is based on DeepFashion1 and DeepFashion2, which are benchmark datasets proposed to study a wide spectrum of computer vision applications for fashion, including online.Automatic facial landmark detection is a longstanding problem in computer vision, and 300-W Challenge is the first event of its kind organized exclusively to benchmark the efforts in the field. 1, 2020 // Host by Challenge data & Generali // Prize: NaN Note : The goal of the challenge is to predict if a building will have an insurance claim during a certain period.
#Death battle snake vs code#
Code will be publicly available at this https URL. Our method also won the 1st place in the DeepFashion2 Challenge 2020 - Clothes Landmark Estimation Track with an AP of 0.590 on the test set, and 0.615 on the validation set. We introduce several powerful post-processing techniques that may be applied to increase the quality of keypoint localization tasks.Extensive experiments show that our method outperforms current state-of-the-art methods by a large margin.
#Death battle snake vs install#
Landmark Estimation Performance on DeepFashion2 Validation Set Quick start Installation Install pytorch >= v1.2 following official instruction.See full list on 点上方蓝字计算机视觉联盟获取更多干货. We won the third place in the "DeepFashion2 Challenge 2020 - Track 1 Clothes Landmark Estimation" competition.
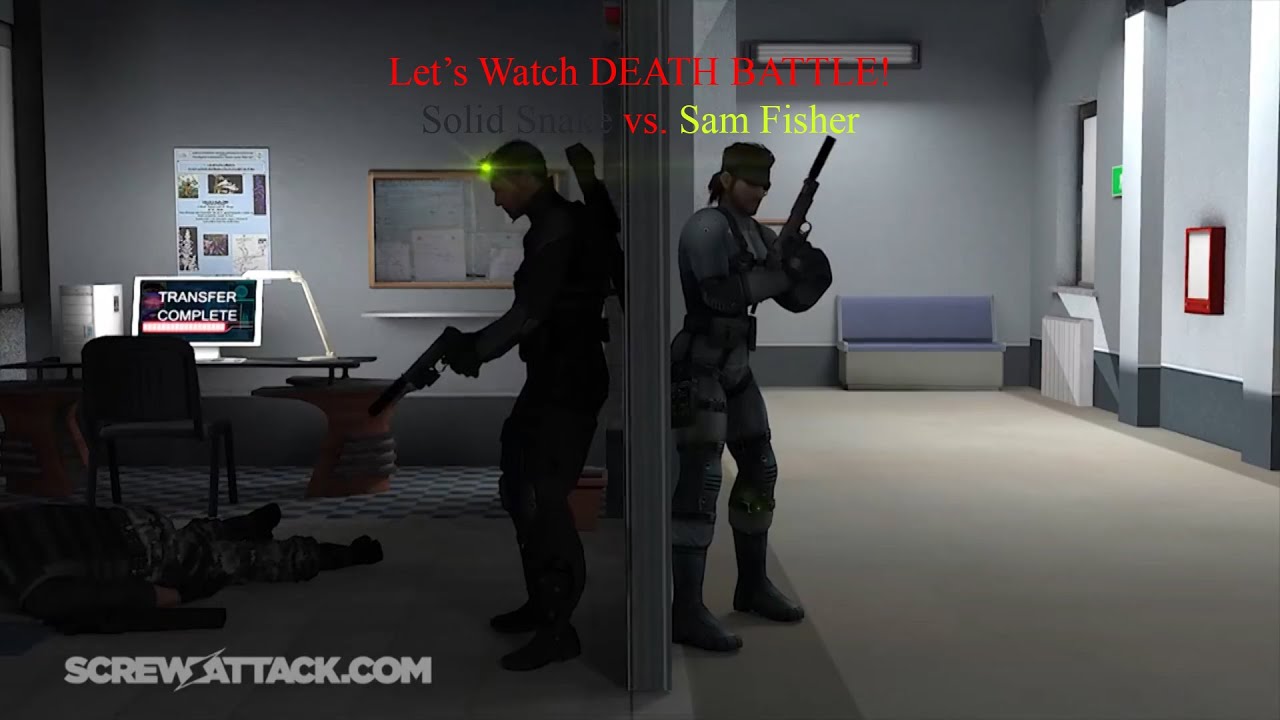
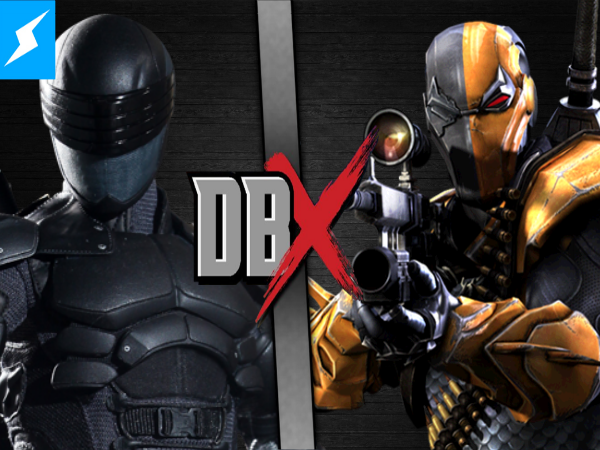
This is not the full dataset as mentioned in, since not all images have been released yet. This dataset contains 191961 images in the train set and 32153 images in the validation set.
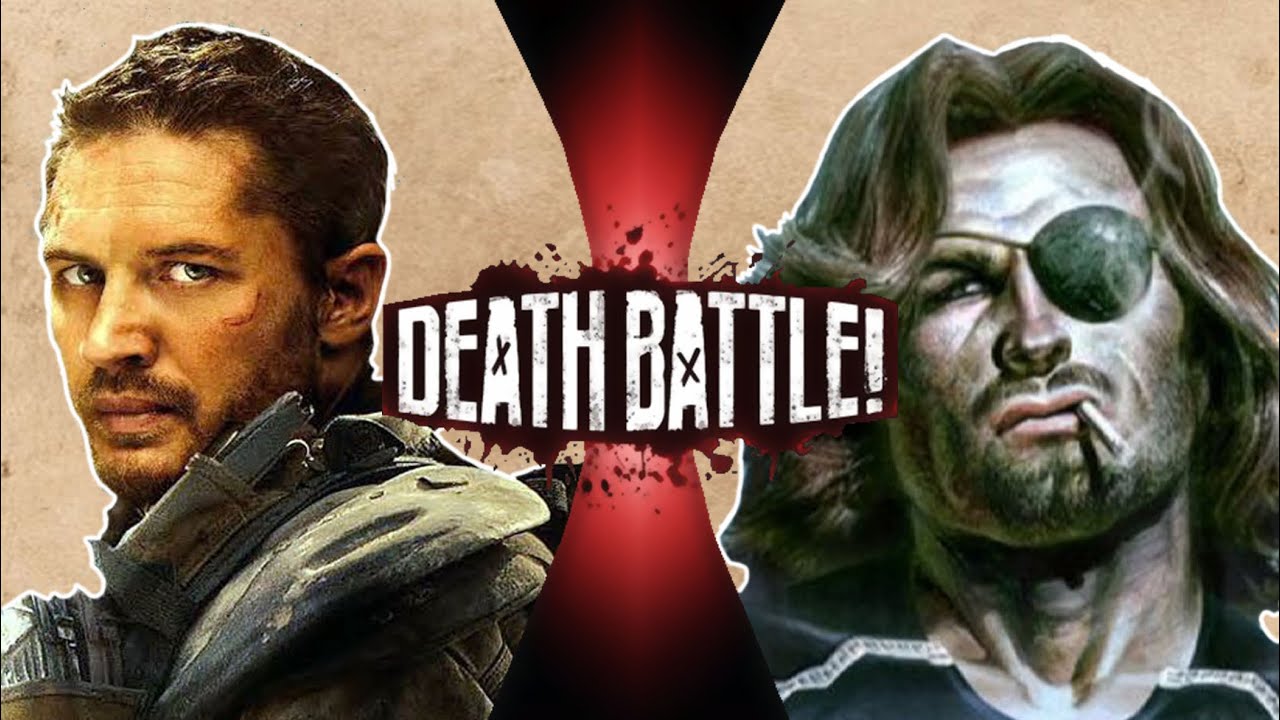
OneDash's new messaging platform allows online shoppers to perform direct, in-app, add-to-cart functions, with the option to check out in the very same chat.For this paper, we use the DeepFashion2 dataset that has been released for the related challenge at ICCV 2019. TORONTO, Ma/PRNewswire-PRWeb/ - OneDash, an e-commerce marketing technology startup today announced that it has launched a groundbreaking chat-to-checkout service. X2 OneDash Launches Innovative Personal Shopping Messenger App.
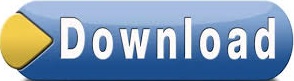